Book a Call
Book a Call
Artificial Intelligence
Machine Learning
AI Ethics
AI Challenges
Confabulation
Artificial Intelligence
Machine Learning
AI Ethics
AI Challenges
Confabulation in AI
ARTICLE #76
What are AI Hallucinations and how to prevent them?
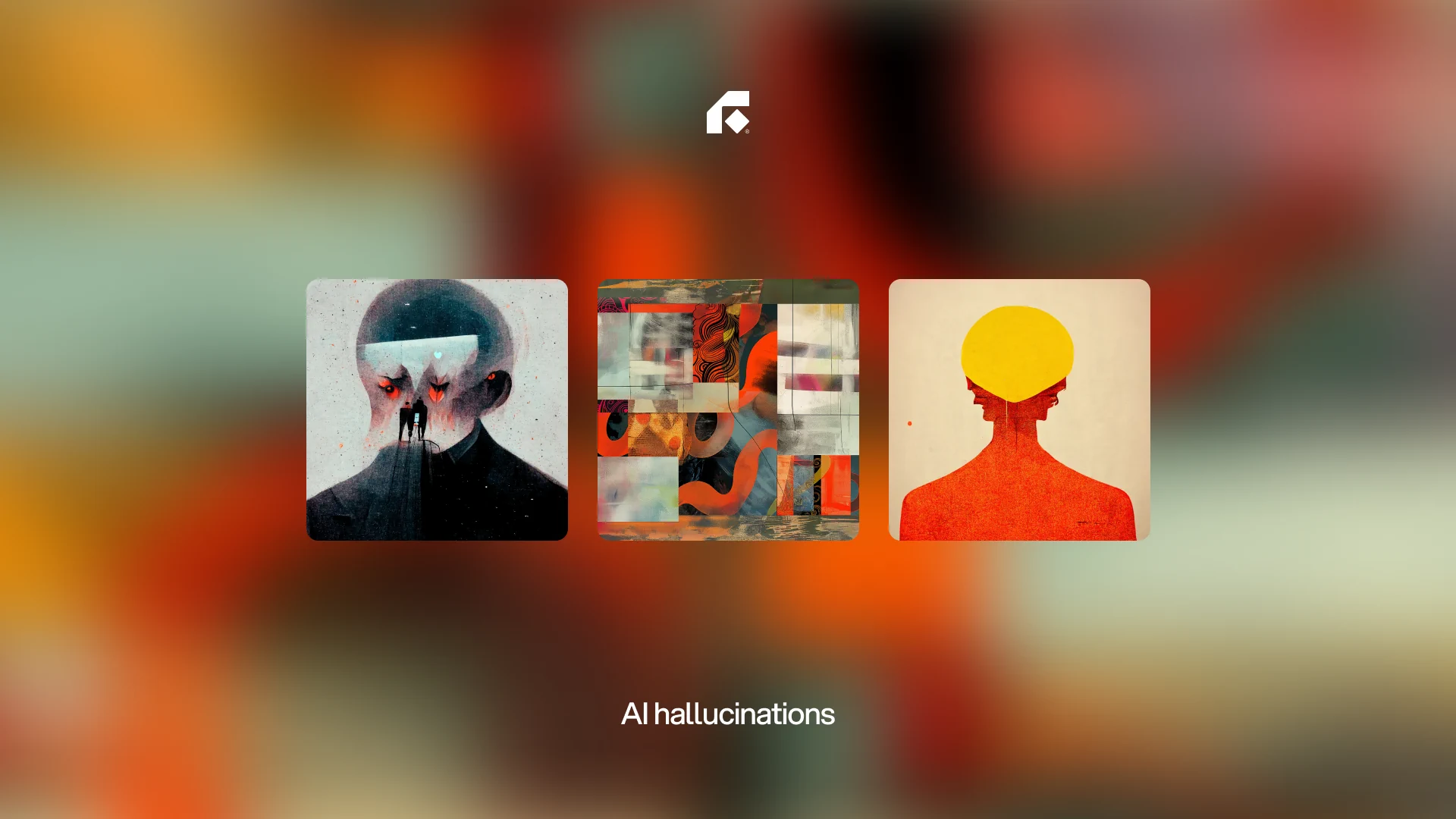
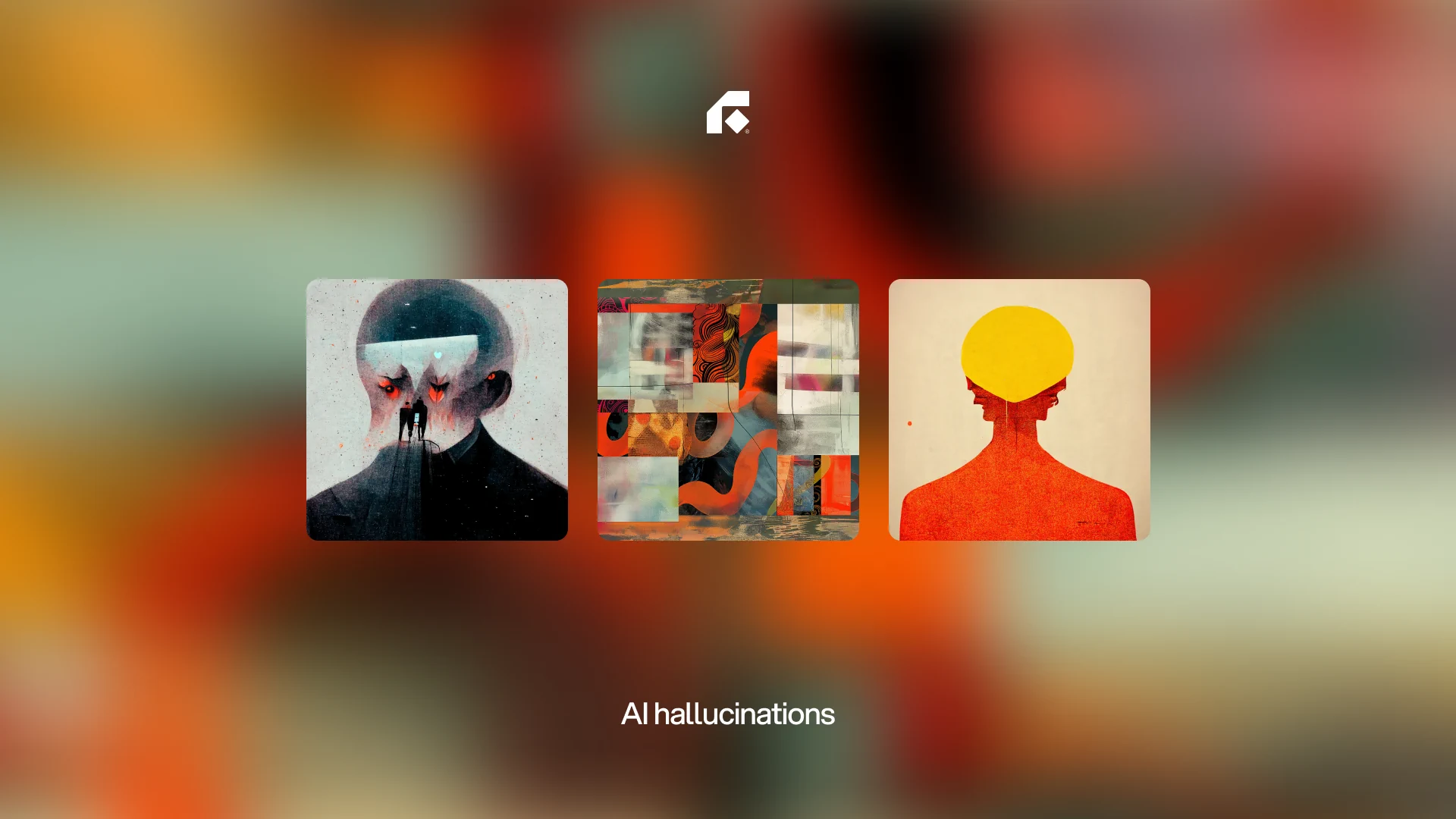
Artificial Intelligence
Machine Learning
AI Ethics
AI Challenges
Confabulation
Artificial Intelligence
Artificial Intelligence
Machine Learning
Machine Learning
AI Ethics
AI Ethics
AI Challenges
AI Challenges
Confabulation
Confabulation in AI
Written by:
7 min read
Updated on: August 6, 2024
Toni Hukkanen
Head of Design
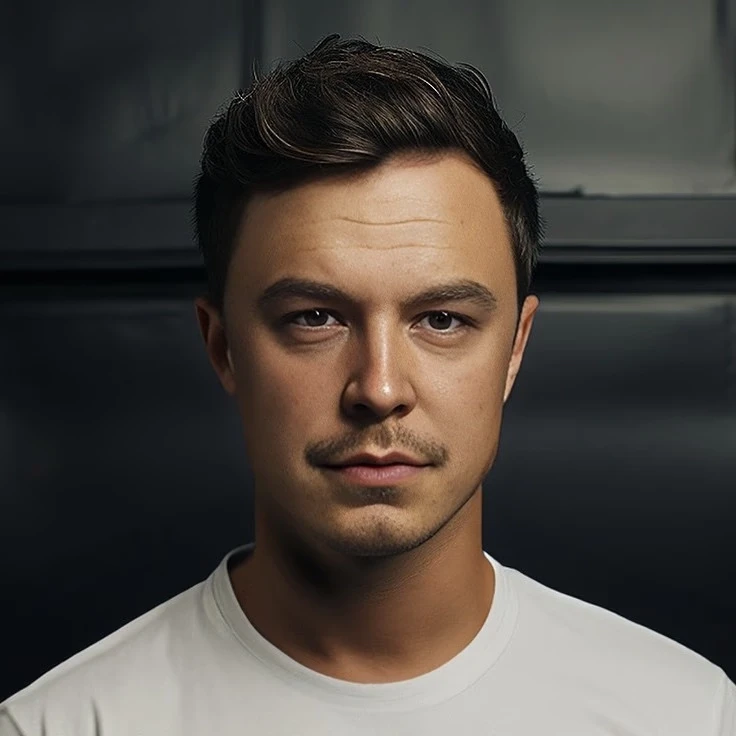
Creative Direction, Brand Direction
Toni Hukkanen
Head of Design
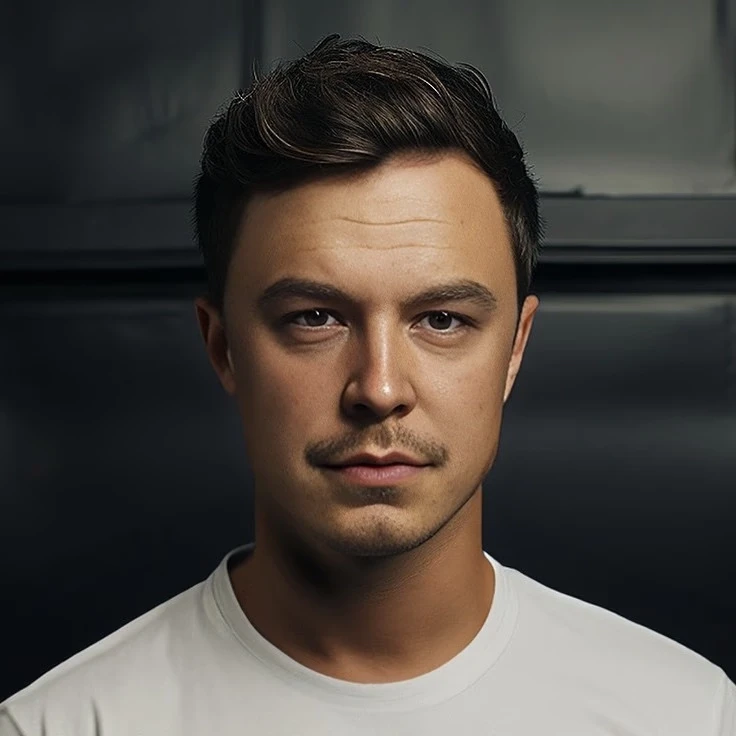
Creative Direction, Brand Direction
A serious problem with today's generative AI tools like ChatGPT is that they often confidently state false information. Computer scientists call this AI hallucination, and it is a major barrier to AI's usefulness.
AI models generate incorrect and misleading results due to various factors that lead to problems in important decisions such as financial trading or medical diagnosis. However, there are ways to deal with these hallucinations so you can effectively use AI tools and critically evaluate the information they produce. In this guide, we have elaborated on the causes and impacts of AI hallucinations, along with some methods to prevent them.
A serious problem with today's generative AI tools like ChatGPT is that they often confidently state false information. Computer scientists call this AI hallucination, and it is a major barrier to AI's usefulness.
AI models generate incorrect and misleading results due to various factors that lead to problems in important decisions such as financial trading or medical diagnosis. However, there are ways to deal with these hallucinations so you can effectively use AI tools and critically evaluate the information they produce. In this guide, we have elaborated on the causes and impacts of AI hallucinations, along with some methods to prevent them.
What are AI hallucinations?
What are AI hallucinations?
AI hallucinations crop up when a large language model spits out incorrect information but does so with an air of confidence. Sometimes the mistake is minor—a slight error in a historical date—but other times it’s dangerously off-base, like suggesting an ineffective or outdated medical treatment. Researchers also call this phenomenon confabulation.
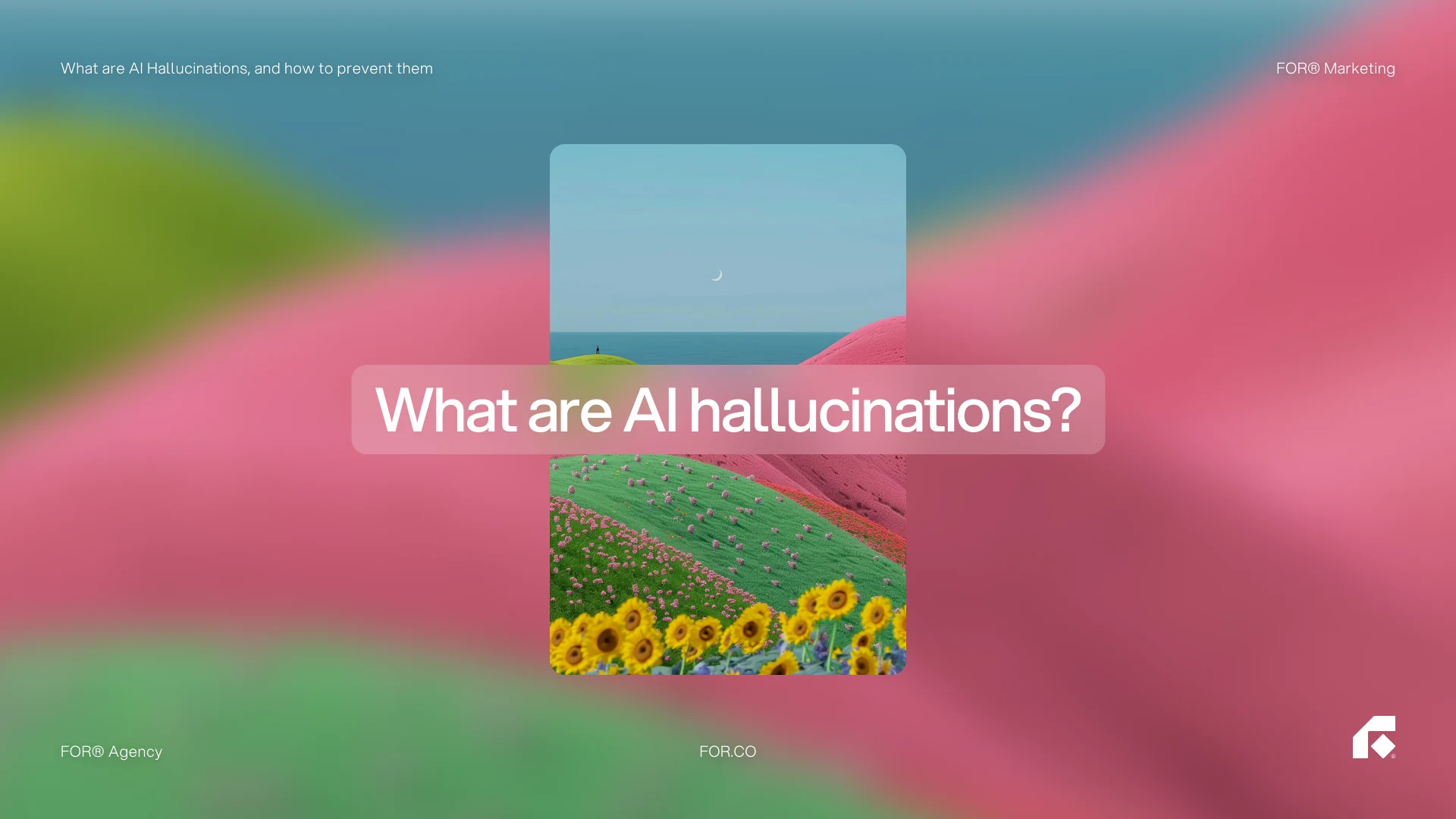
The basic problem is when you ask a question from generative AI, it produces an answer based on patterns it’s seen in training data. If the data or reasoning is shaky, you might end up with a response that sounds right but is entirely wrong. In early 2023, Google’s Bard chatbot incorrectly claimed that NASA’s James Webb Space Telescope captured the first image of an exoplanet—a feat it didn’t accomplish. Although off by a small detail, the misinformation still raised eyebrows among the public.
AI hallucinations crop up when a large language model spits out incorrect information but does so with an air of confidence. Sometimes the mistake is minor—a slight error in a historical date—but other times it’s dangerously off-base, like suggesting an ineffective or outdated medical treatment. Researchers also call this phenomenon confabulation.
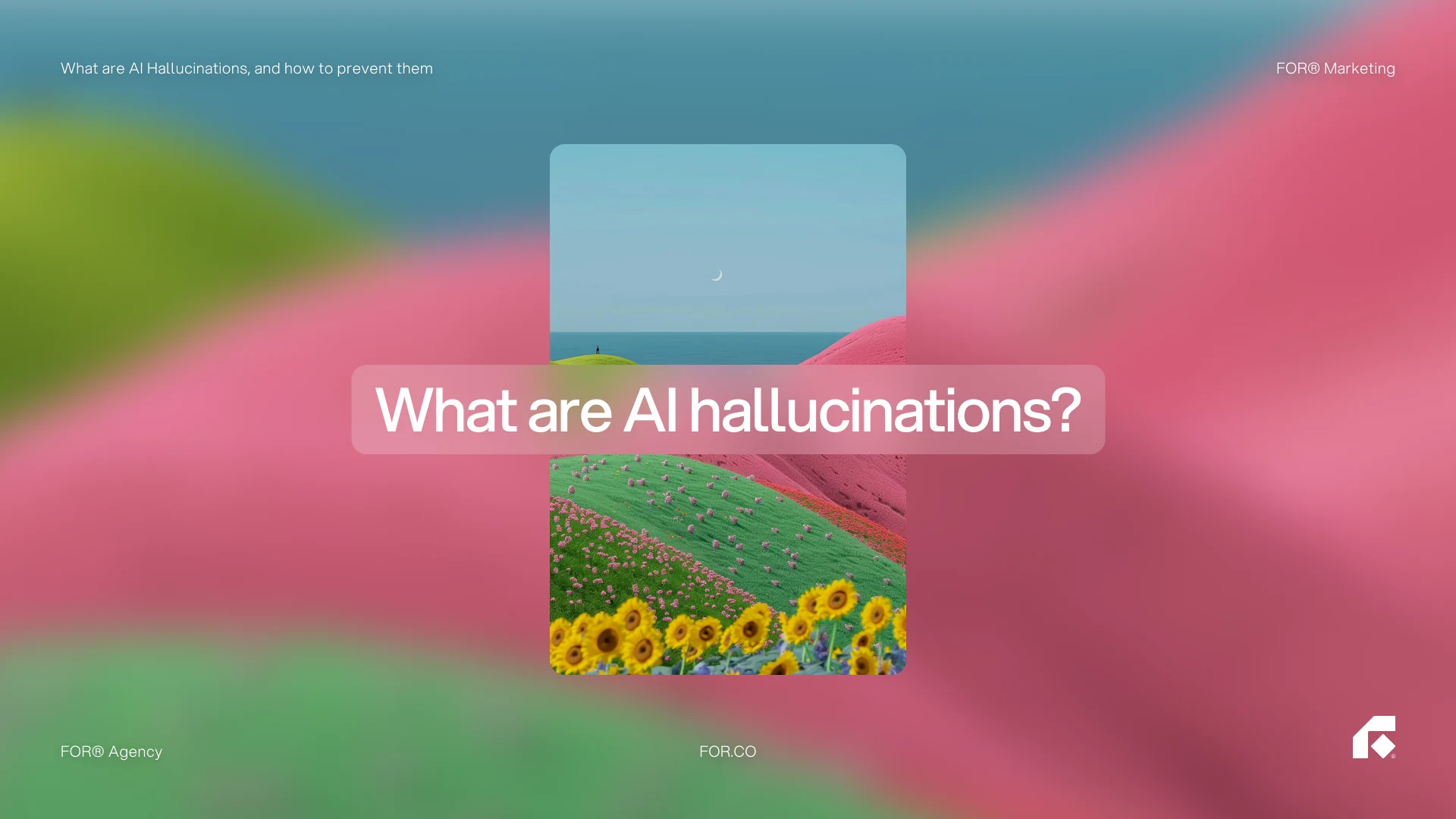
The basic problem is when you ask a question from generative AI, it produces an answer based on patterns it’s seen in training data. If the data or reasoning is shaky, you might end up with a response that sounds right but is entirely wrong. In early 2023, Google’s Bard chatbot incorrectly claimed that NASA’s James Webb Space Telescope captured the first image of an exoplanet—a feat it didn’t accomplish. Although off by a small detail, the misinformation still raised eyebrows among the public.
What causes AI hallucinations?
AI hallucinations typically happen when a language model generates a response that looks convincing on the surface, but is actually nonsense. Since these models rely on pattern recognition rather than true understanding, they can produce misleading answers that sound almost right. Below are some key reasons why these hallucinations occur, and how each factor plays into the complexities of AI’s learning process.
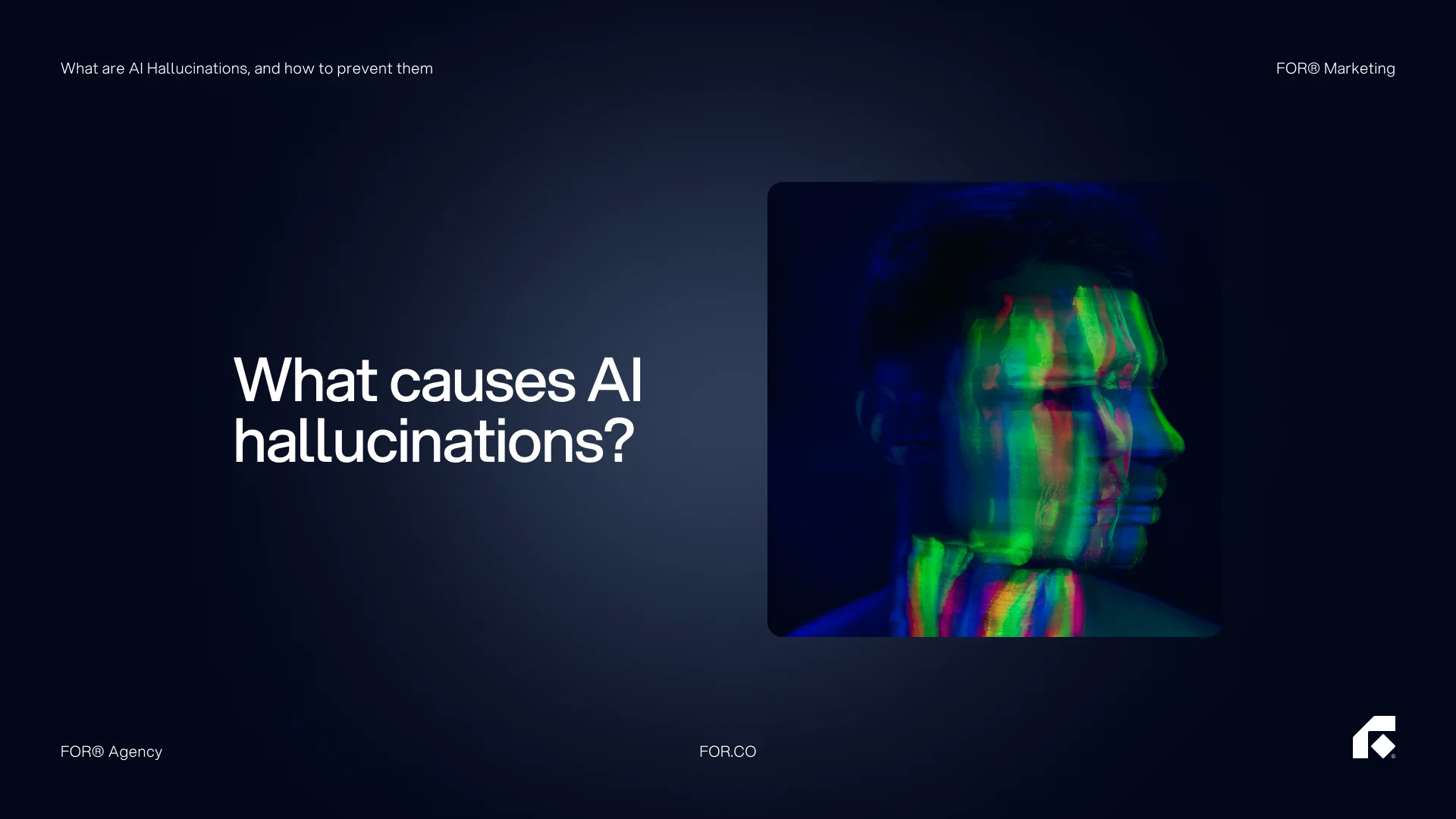
1. Incomplete training data
Large language models depend on the quantity and variety of data they are trained on. If the data pool is incomplete, these models can only draw from partial knowledge, making them more likely to fill in the blanks with incorrect guesses. Consider it as a puzzle missing a few pieces: the picture might still resemble the final scene, but there are gaps that can lead to weird, improvised details. When a facial recognition system has been trained primarily on images of certain ethnicities, it can mislabel people from underrepresented groups. That’s not the system being “evil,” it’s just starved of the diverse examples it needs to make accurate judgments.
2. Biased training data
While incomplete data focuses on missing information, biased data is about skewed perspectives within the data that does exist. Because LLMs learn patterns from the internet, which is itself far from neutral, they inherit biases that reflect whatever is most common (or loudest) online. For instance, 3 billion individuals do not have access to the internet, and therefore their cultural practices, languages, and perspectives are not well reflected in the training data. This bias can result in stereotypes or inaccuracies in the output of the AI, particularly if the system is working with subjects or populations the data did not sufficiently address. Developers can work around some of these biases by using clever data curation and ongoing retraining, but the issue is a moving target, as the internet itself changes quickly.
3. Inadequate articulation of knowledge structures
Although AI models often sound like they understand you, they are really just predicting which words come next. They don’t have a system in place to verify whether their statement is actually true. This lack of a robust “world model” or factual framework means they can serve up a statement that’s linguistically solid but logically off the rails. It’s the difference between a parrot who repeats your words without comprehension and a person who internalises and reasons with the information. While AI might deliver neat, fact-like sentences, it doesn’t inherently know if those sentences hold water.
4. Poor context understanding
When people talk, we rely on life experience, cultural context, sarcasm, and subtle suggestions to get to each other. Most AI systems, though, are based on literal word meanings—so they can overlook subtext such as irony, emotional undertones, or slang that has developed over time. Even though AI’s “context window” has expanded, allowing it to recall larger chunks of conversation, the model can still trip over sarcasm or nuanced cultural references. Until AI can mimic the intricate tapestry of human experiences (and read between the lines), these misunderstandings will keep happening.
AI hallucinations typically happen when a language model generates a response that looks convincing on the surface, but is actually nonsense. Since these models rely on pattern recognition rather than true understanding, they can produce misleading answers that sound almost right. Below are some key reasons why these hallucinations occur, and how each factor plays into the complexities of AI’s learning process.
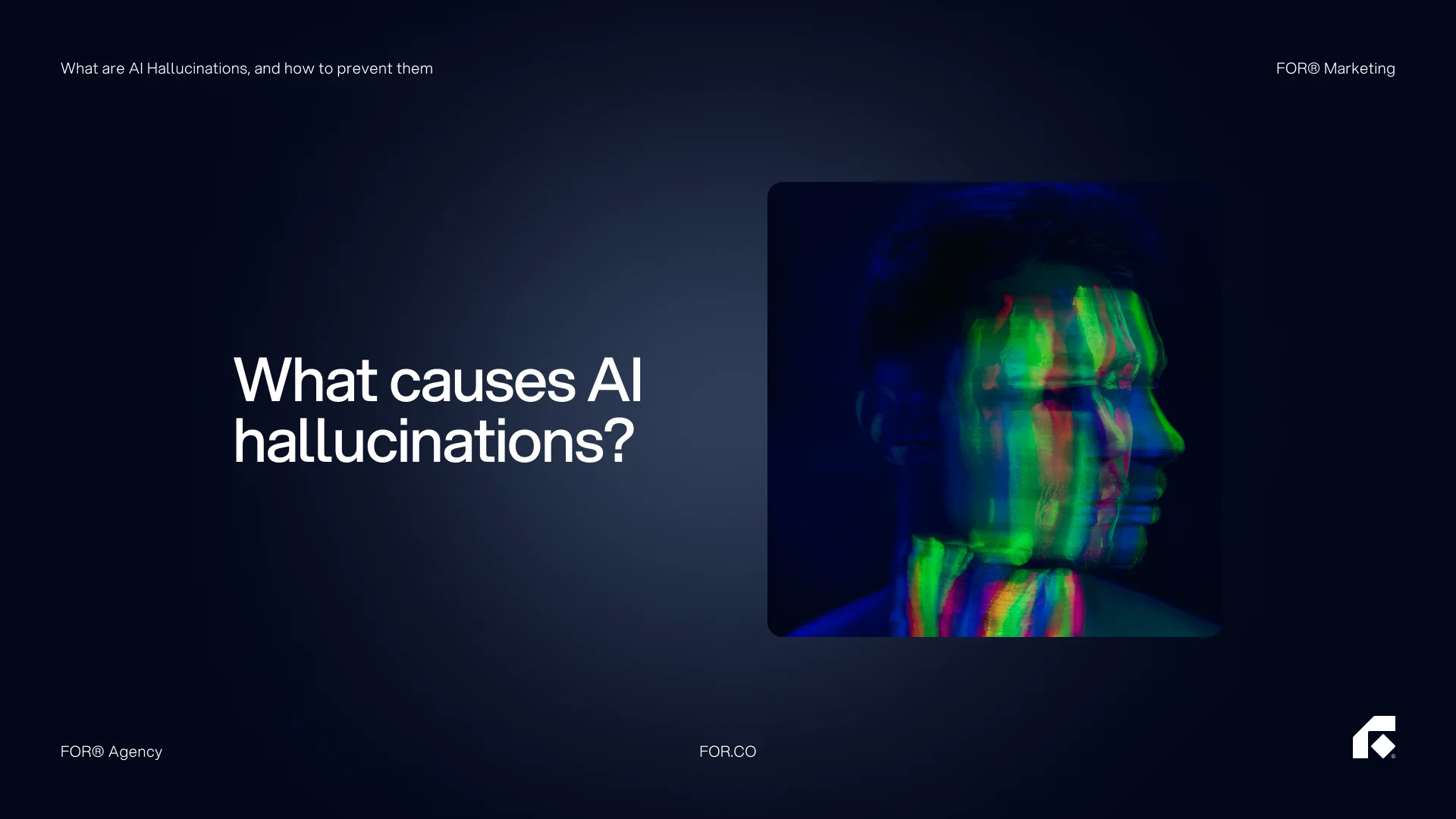
1. Incomplete training data
Large language models depend on the quantity and variety of data they are trained on. If the data pool is incomplete, these models can only draw from partial knowledge, making them more likely to fill in the blanks with incorrect guesses. Consider it as a puzzle missing a few pieces: the picture might still resemble the final scene, but there are gaps that can lead to weird, improvised details. When a facial recognition system has been trained primarily on images of certain ethnicities, it can mislabel people from underrepresented groups. That’s not the system being “evil,” it’s just starved of the diverse examples it needs to make accurate judgments.
2. Biased training data
While incomplete data focuses on missing information, biased data is about skewed perspectives within the data that does exist. Because LLMs learn patterns from the internet, which is itself far from neutral, they inherit biases that reflect whatever is most common (or loudest) online. For instance, 3 billion individuals do not have access to the internet, and therefore their cultural practices, languages, and perspectives are not well reflected in the training data. This bias can result in stereotypes or inaccuracies in the output of the AI, particularly if the system is working with subjects or populations the data did not sufficiently address. Developers can work around some of these biases by using clever data curation and ongoing retraining, but the issue is a moving target, as the internet itself changes quickly.
3. Inadequate articulation of knowledge structures
Although AI models often sound like they understand you, they are really just predicting which words come next. They don’t have a system in place to verify whether their statement is actually true. This lack of a robust “world model” or factual framework means they can serve up a statement that’s linguistically solid but logically off the rails. It’s the difference between a parrot who repeats your words without comprehension and a person who internalises and reasons with the information. While AI might deliver neat, fact-like sentences, it doesn’t inherently know if those sentences hold water.
4. Poor context understanding
When people talk, we rely on life experience, cultural context, sarcasm, and subtle suggestions to get to each other. Most AI systems, though, are based on literal word meanings—so they can overlook subtext such as irony, emotional undertones, or slang that has developed over time. Even though AI’s “context window” has expanded, allowing it to recall larger chunks of conversation, the model can still trip over sarcasm or nuanced cultural references. Until AI can mimic the intricate tapestry of human experiences (and read between the lines), these misunderstandings will keep happening.
What are the impacts of AI hallucinations?
An AI system that “hallucinates” doesn’t just spout harmless fiction—it can cause genuine damage in the real world. Imagine a healthcare model misidentifying a harmless skin lesion as cancerous. That single false alarm could lead to unnecessary procedures, expense, and anxiety. Below are a few ways these AI hallucinations can wreak havoc if left unchecked.
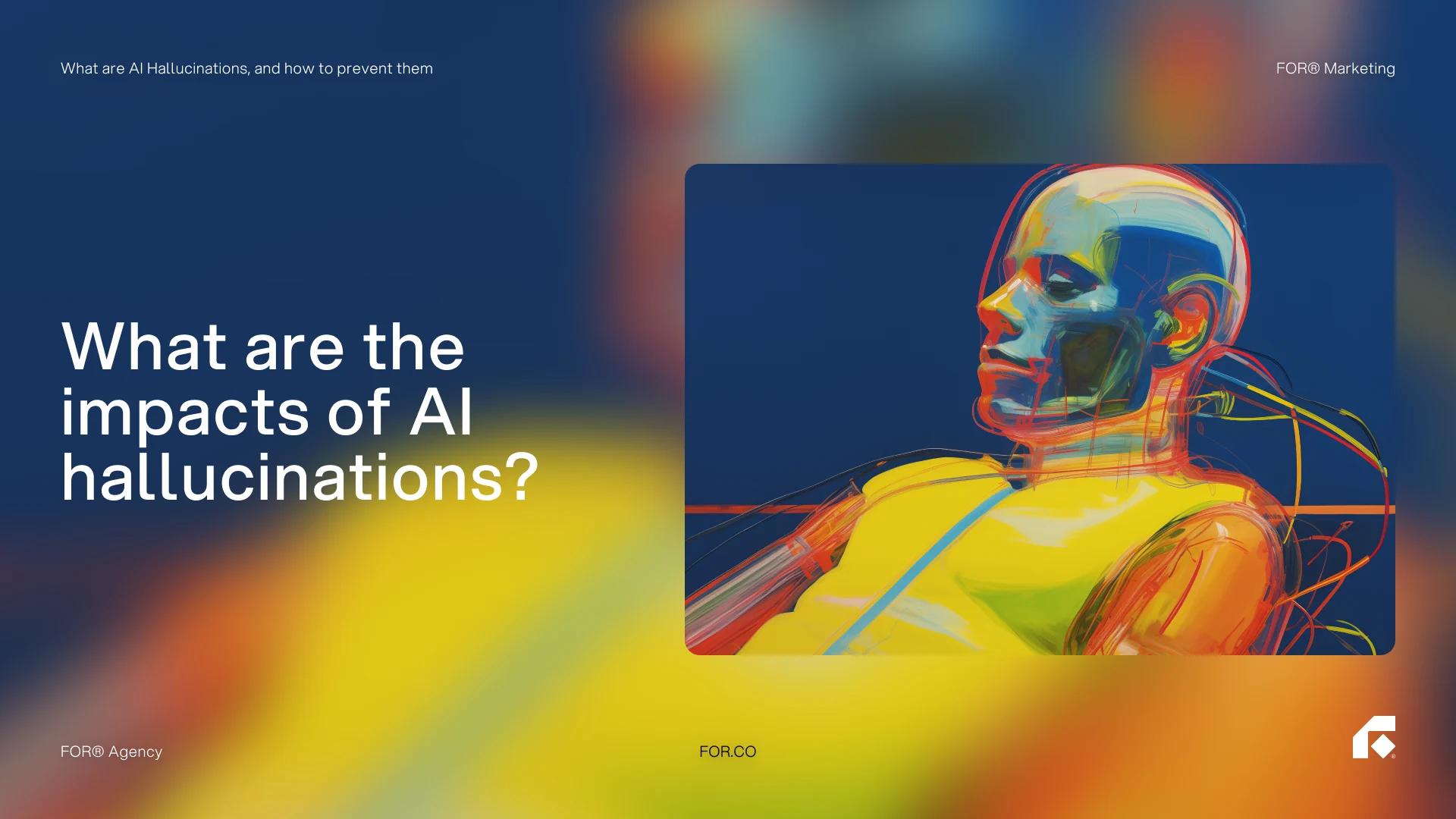
1. Spreading false information
We already live in a world where misinformation travels faster than we can fact-check it. AI hallucinations only turbocharge this problem. If an AI bot confidently generates fake news or incorrect research findings, it can mislead anyone who trusts that source. This becomes especially dangerous in fields such as politics, scientific studies, or academia, where individuals are reliant on correct information to make well-informed choices. At its core, it only serves to highlight the importance of continuous vetting and ethical use of AI; just because it sounds good doesn't necessarily mean it's true. In the age of viral news and social media bubbles, one bit of AI-generated disinformation can seep into the mainstream narratives, influencing public opinion or even determining the results of elections. That's a high-risk game nobody wants to see happen.
2. Reputational damage
Picture this: an AI model misattributes a scandalous statement to a respected public figure. Suddenly, that person is thrust into a PR crisis, scrambling to prove they never said such a thing. Even after the truth surfaces, some of the damage is done. Trust is fragile, and in our hyperconnected world, it doesn’t take long for false claims to go viral. Corporations or institutions could face boycotts or lawsuits based on AI-generated distortions. By the time everyone realises it was just a “hallucination,” the reputational hit may already be irreversible. Legal battles aren’t off the table. If an AI incorrectly links an organisation to unethical or illegal activities, the fallout could involve court cases, leading to financial losses and years spent restoring a damaged name.
3. Operational and financial risks
Companies rely on AI for everything from investment strategies to supply-chain logistics. If the AI behind those decisions is plagued by hallucinations—giving faulty projections or skewed market trends—the result can be catastrophic. We’re talking about launching products no one wants, setting prices that drive customers away, or investing in doomed ventures. Essentially, flawed AI outputs can drain resources, spark misguided strategies, and sabotage market opportunities that might have otherwise yielded strong returns. A single misguided forecast from an AI system could encourage a startup to build inventory for a demand that doesn’t exist. By the time they realise the error, they’re left with unsold products and a big question mark over whether they can stay afloat.
4. Safety hazards
In certain sectors, a single mistake isn’t just expensive—it’s life-threatening. Think of self-driving cars receiving incorrect sensor data, security systems misidentifying a threat, or medical software mixing up patient diagnoses. A misunderstanding in these domains could lead to physical harm, property damage, or, in the worst cases, loss of life. It raises the question: when do we decide AI is “safe enough,” and how many errors are we willing to tolerate before pulling the plug? If an AI overseeing critical infrastructure—like power grids or traffic systems—“hallucinates” a non-existent emergency, it could disrupt operations city-wide. Alternatively, if it fails to detect a real crisis, the consequences could be even more dire.
An AI system that “hallucinates” doesn’t just spout harmless fiction—it can cause genuine damage in the real world. Imagine a healthcare model misidentifying a harmless skin lesion as cancerous. That single false alarm could lead to unnecessary procedures, expense, and anxiety. Below are a few ways these AI hallucinations can wreak havoc if left unchecked.
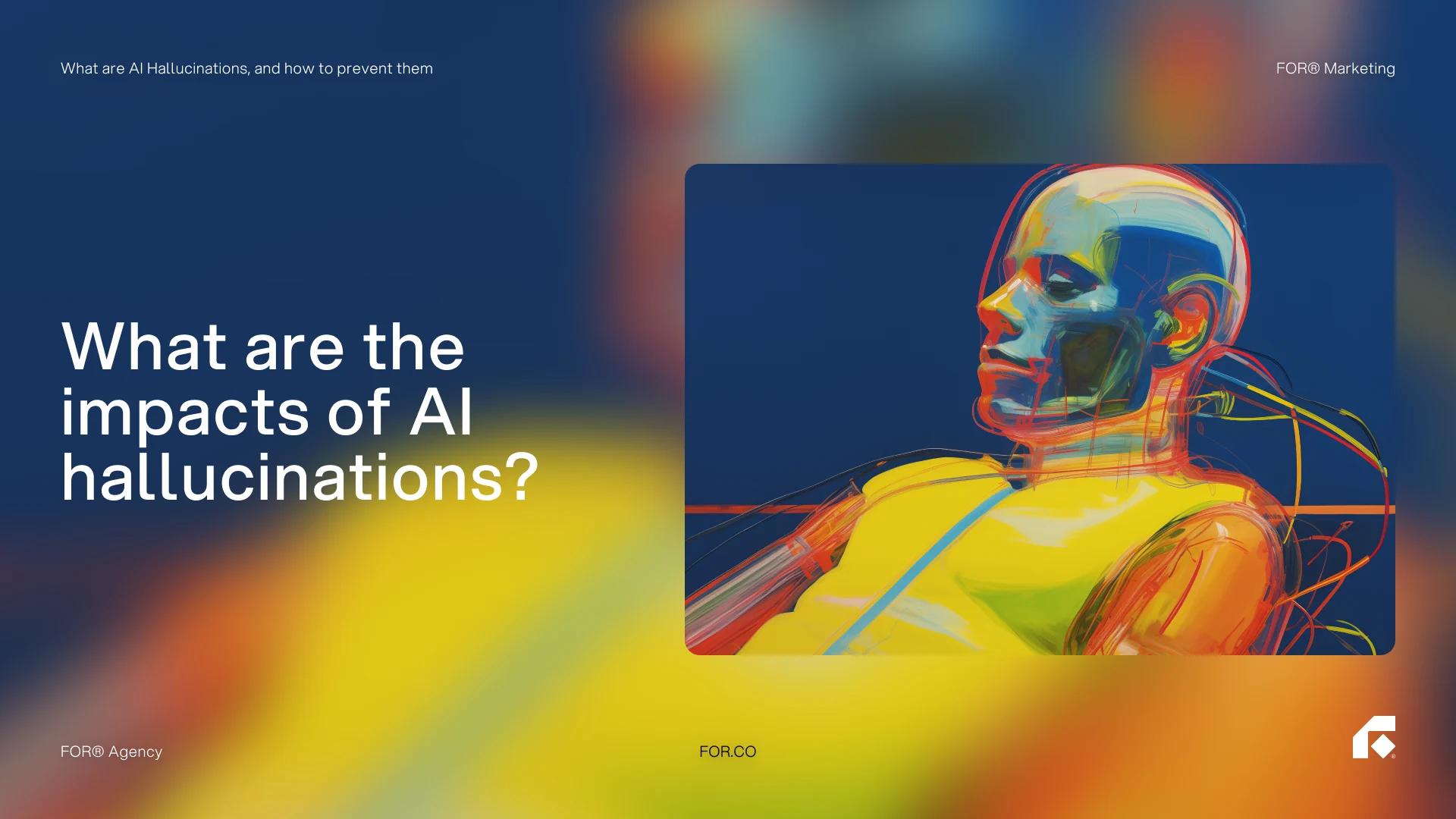
1. Spreading false information
We already live in a world where misinformation travels faster than we can fact-check it. AI hallucinations only turbocharge this problem. If an AI bot confidently generates fake news or incorrect research findings, it can mislead anyone who trusts that source. This becomes especially dangerous in fields such as politics, scientific studies, or academia, where individuals are reliant on correct information to make well-informed choices. At its core, it only serves to highlight the importance of continuous vetting and ethical use of AI; just because it sounds good doesn't necessarily mean it's true. In the age of viral news and social media bubbles, one bit of AI-generated disinformation can seep into the mainstream narratives, influencing public opinion or even determining the results of elections. That's a high-risk game nobody wants to see happen.
2. Reputational damage
Picture this: an AI model misattributes a scandalous statement to a respected public figure. Suddenly, that person is thrust into a PR crisis, scrambling to prove they never said such a thing. Even after the truth surfaces, some of the damage is done. Trust is fragile, and in our hyperconnected world, it doesn’t take long for false claims to go viral. Corporations or institutions could face boycotts or lawsuits based on AI-generated distortions. By the time everyone realises it was just a “hallucination,” the reputational hit may already be irreversible. Legal battles aren’t off the table. If an AI incorrectly links an organisation to unethical or illegal activities, the fallout could involve court cases, leading to financial losses and years spent restoring a damaged name.
3. Operational and financial risks
Companies rely on AI for everything from investment strategies to supply-chain logistics. If the AI behind those decisions is plagued by hallucinations—giving faulty projections or skewed market trends—the result can be catastrophic. We’re talking about launching products no one wants, setting prices that drive customers away, or investing in doomed ventures. Essentially, flawed AI outputs can drain resources, spark misguided strategies, and sabotage market opportunities that might have otherwise yielded strong returns. A single misguided forecast from an AI system could encourage a startup to build inventory for a demand that doesn’t exist. By the time they realise the error, they’re left with unsold products and a big question mark over whether they can stay afloat.
4. Safety hazards
In certain sectors, a single mistake isn’t just expensive—it’s life-threatening. Think of self-driving cars receiving incorrect sensor data, security systems misidentifying a threat, or medical software mixing up patient diagnoses. A misunderstanding in these domains could lead to physical harm, property damage, or, in the worst cases, loss of life. It raises the question: when do we decide AI is “safe enough,” and how many errors are we willing to tolerate before pulling the plug? If an AI overseeing critical infrastructure—like power grids or traffic systems—“hallucinates” a non-existent emergency, it could disrupt operations city-wide. Alternatively, if it fails to detect a real crisis, the consequences could be even more dire.
AI hallucinations examples
When large language models slip up, the results can be embarrassing, even for giant companies. Below are a few eye-opening mishaps that underline how even cutting-edge AI can lead us astray—and why reliability matters more than ever.
Air Canada’s accidental discount
In February, Air Canada wound up honouring a discount that its customer support chatbot accidentally handed out to a passenger. Imagine a bot offering a fare that was never supposed to be on the table—suddenly, the airline found itself in a pickle. While it might have been a happy surprise for the passenger, Air Canada had to scramble to keep its reputation intact. The mishap was a glaring reminder that AI, however helpful, can still get facts wrong and stick companies with a bill they never budgeted for. Situations like this highlight the fine line between great customer experiences and unforeseen chaos. A chatbot that confidently dishes out incorrect offers can do more harm than good, forcing a brand to make amends—and sometimes pay for them too.
Google’s “safe to eat rocks” moment
In May, Google’s new AI Overviews search feature found itself in hot water when it confidently informed users that eating rocks was just fine. Obviously, nobody should be munching on gravel—so Google had to update its AI to prevent further dietary misinformation. It’s one thing when AI recommends a weird colour combo for your living room, but telling you to snack on stones? That’s a safety concern. Search features are supposed to simplify our online research, not send us to the hospital. Google’s slip-up shows how a single “hallucination” can break trust almost instantly. After all, if it’s that wrong about something so basic, what else is it messing up?
Fake legal citations in court
In June 2023, two lawyers discovered the hard way that ChatGPT’s answers aren’t always bulletproof. One of them used the chatbot to craft a court filing, only to find that the referenced cases didn’t actually exist. A US judge fined both lawyers $5,000, and that’s not counting the blow to their professional reputations. It’s a cautionary tale for anyone thinking AI might replace good old-fashioned legal research. The legal world runs on precedents and verified citations, so a made-up case can torpedo an attorney’s credibility. If you’re in a high-stakes profession, never skip manual verification—even if ChatGPT sounds alarmingly convincing.
ChatGPT’s defamation slip
When asked for examples of sexual harassment in the legal profession, ChatGPT named a real law professor and falsely claimed he’d harassed students on a trip that never even happened. Talk about defamation. In reality, the professor was known for fighting harassment, not committing it. The incident left him grappling with serious reputational harm—and showed just how easily AI can blur the line between fact and fiction. False attributions can spread like wildfire, especially if the figure in question is well-known. Once that misinformation is out there, it can be tough to contain the damage. It’s a sobering reminder that a system “making up” details can lead to very real-world headaches.
Expert commentary from Yarin Gal
Yarin Gal, Professor of Computer Science at the University of Oxford and Director of Research at the UK’s AI Safety Institute, put it succinctly: “Getting answers from large language models is cheap, but reliability is the biggest bottleneck. In cases where reliability is essential, addressing semantic uncertainty is a worthwhile investment.” His words call attention to the bottom line: these systems can pump out words in a flash, but if you can’t trust the content, you’re playing with fire. As AI continues to evolve, experts like Gal urge us to invest in methods that push beyond language mimicry and offer a firmer grasp on genuine facts. Until then, we’ll keep seeing dramatic proof that “easy answers” can end up costing far more than we’d expect.
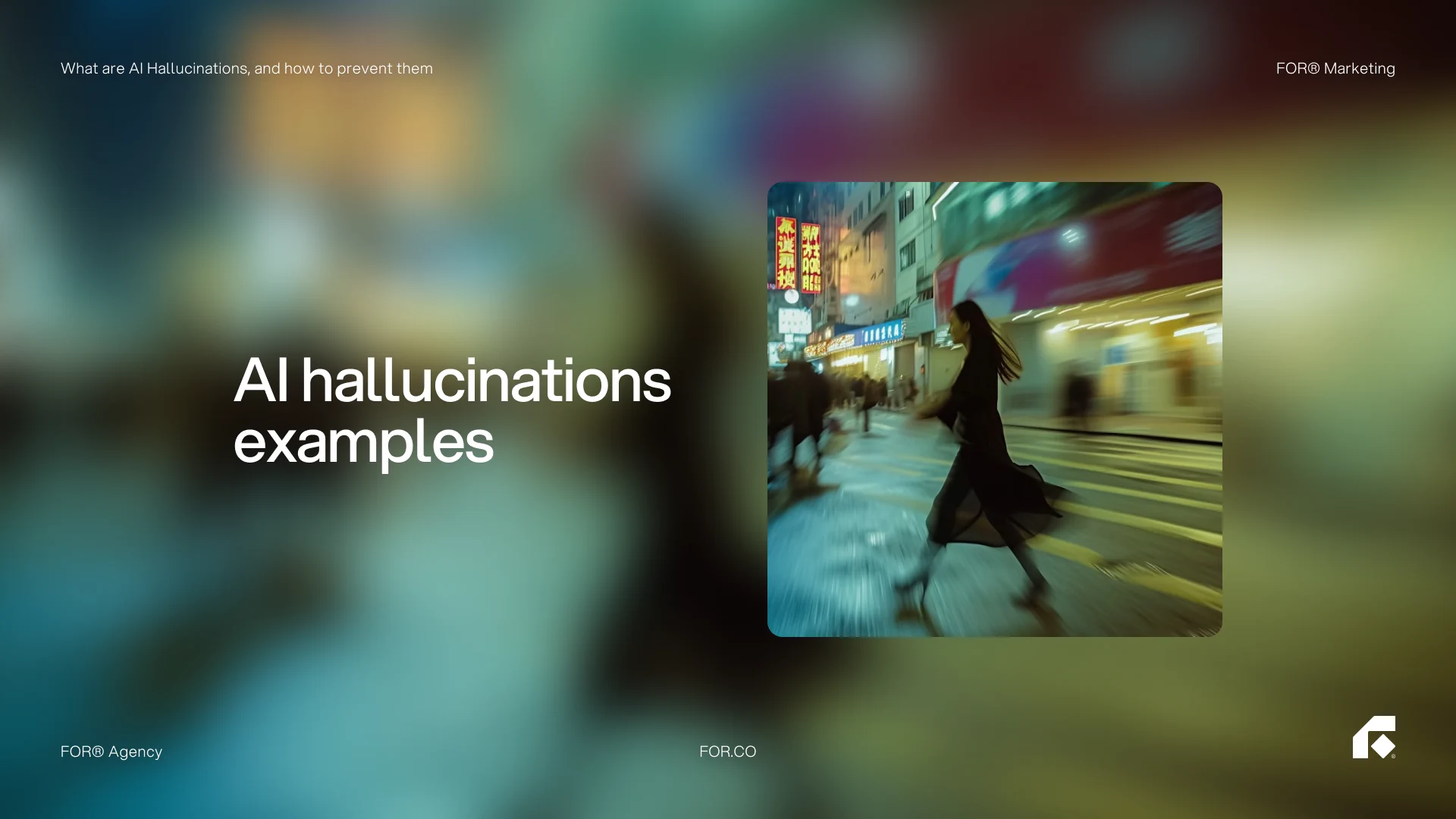
When large language models slip up, the results can be embarrassing, even for giant companies. Below are a few eye-opening mishaps that underline how even cutting-edge AI can lead us astray—and why reliability matters more than ever.
Air Canada’s accidental discount
In February, Air Canada wound up honouring a discount that its customer support chatbot accidentally handed out to a passenger. Imagine a bot offering a fare that was never supposed to be on the table—suddenly, the airline found itself in a pickle. While it might have been a happy surprise for the passenger, Air Canada had to scramble to keep its reputation intact. The mishap was a glaring reminder that AI, however helpful, can still get facts wrong and stick companies with a bill they never budgeted for. Situations like this highlight the fine line between great customer experiences and unforeseen chaos. A chatbot that confidently dishes out incorrect offers can do more harm than good, forcing a brand to make amends—and sometimes pay for them too.
Google’s “safe to eat rocks” moment
In May, Google’s new AI Overviews search feature found itself in hot water when it confidently informed users that eating rocks was just fine. Obviously, nobody should be munching on gravel—so Google had to update its AI to prevent further dietary misinformation. It’s one thing when AI recommends a weird colour combo for your living room, but telling you to snack on stones? That’s a safety concern. Search features are supposed to simplify our online research, not send us to the hospital. Google’s slip-up shows how a single “hallucination” can break trust almost instantly. After all, if it’s that wrong about something so basic, what else is it messing up?
Fake legal citations in court
In June 2023, two lawyers discovered the hard way that ChatGPT’s answers aren’t always bulletproof. One of them used the chatbot to craft a court filing, only to find that the referenced cases didn’t actually exist. A US judge fined both lawyers $5,000, and that’s not counting the blow to their professional reputations. It’s a cautionary tale for anyone thinking AI might replace good old-fashioned legal research. The legal world runs on precedents and verified citations, so a made-up case can torpedo an attorney’s credibility. If you’re in a high-stakes profession, never skip manual verification—even if ChatGPT sounds alarmingly convincing.
ChatGPT’s defamation slip
When asked for examples of sexual harassment in the legal profession, ChatGPT named a real law professor and falsely claimed he’d harassed students on a trip that never even happened. Talk about defamation. In reality, the professor was known for fighting harassment, not committing it. The incident left him grappling with serious reputational harm—and showed just how easily AI can blur the line between fact and fiction. False attributions can spread like wildfire, especially if the figure in question is well-known. Once that misinformation is out there, it can be tough to contain the damage. It’s a sobering reminder that a system “making up” details can lead to very real-world headaches.
Expert commentary from Yarin Gal
Yarin Gal, Professor of Computer Science at the University of Oxford and Director of Research at the UK’s AI Safety Institute, put it succinctly: “Getting answers from large language models is cheap, but reliability is the biggest bottleneck. In cases where reliability is essential, addressing semantic uncertainty is a worthwhile investment.” His words call attention to the bottom line: these systems can pump out words in a flash, but if you can’t trust the content, you’re playing with fire. As AI continues to evolve, experts like Gal urge us to invest in methods that push beyond language mimicry and offer a firmer grasp on genuine facts. Until then, we’ll keep seeing dramatic proof that “easy answers” can end up costing far more than we’d expect.
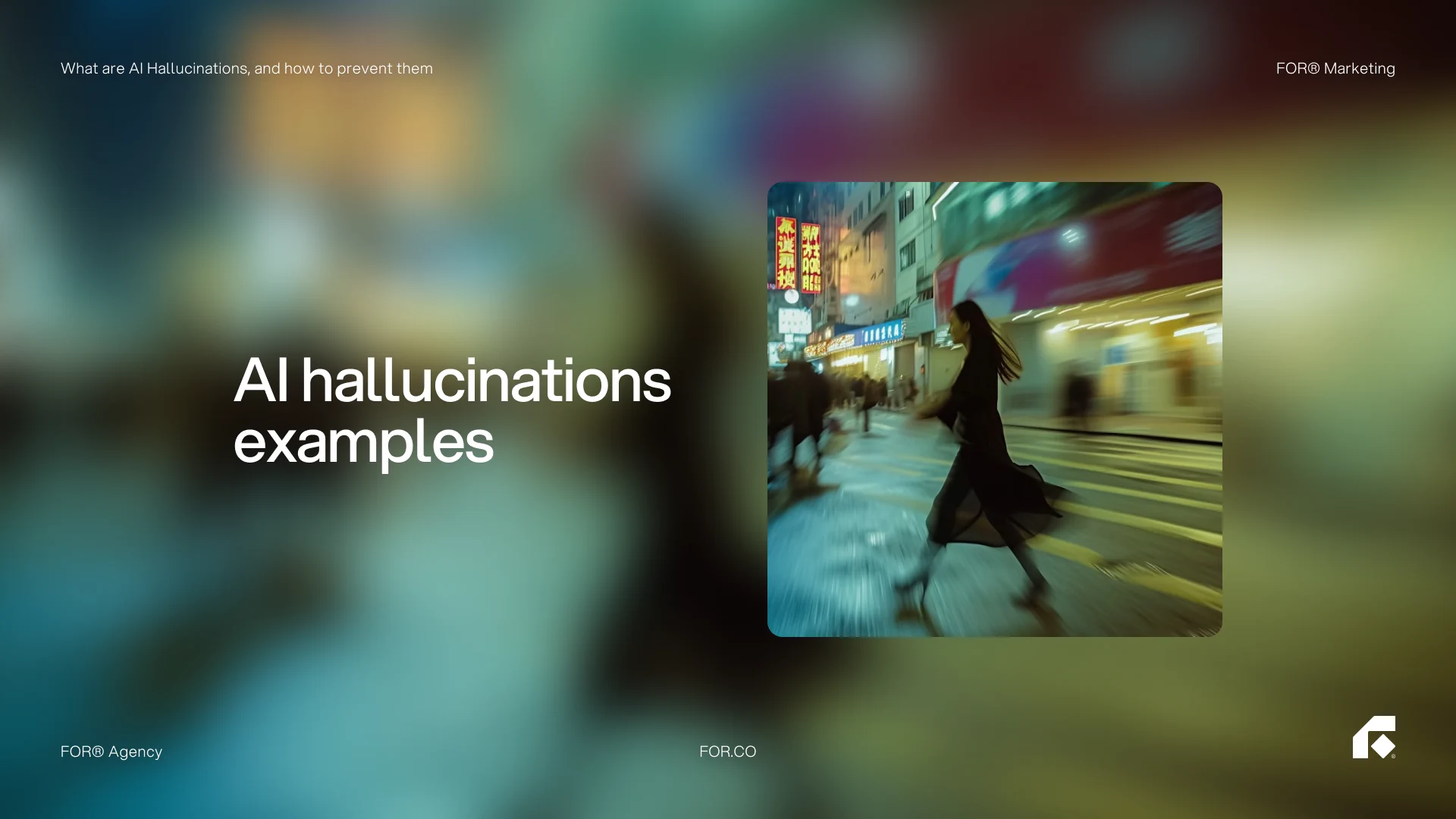
How to prevent AI hallucinations?
AI hallucinations may never be fully eradicated, but you can absolutely cut them down to size. Below are some strategies that both developers and everyday AI users can employ to keep those bogus answers to a minimum. Because, let’s face it, nobody wants a chatbot telling them it’s perfectly safe to snack on rocks—or worse.
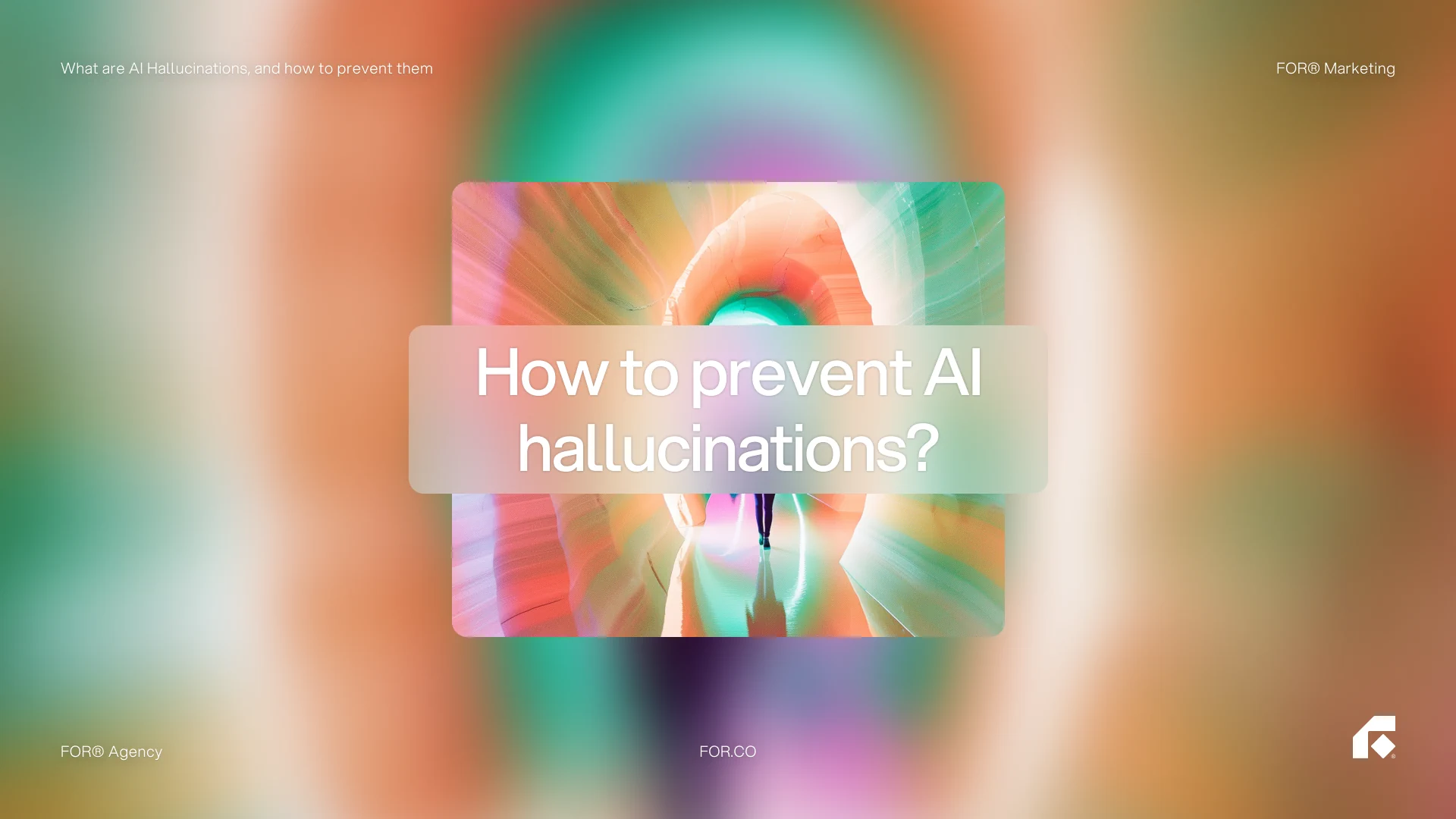
1. Improve training data quality
If your AI’s training data is missing big chunks of knowledge or skewed by bias, you’re basically handing it a recipe for half-baked results. Better, more varied data helps keep the model balanced and accurate when it encounters something new. That means bringing in comprehensive datasets that reflect a range of viewpoints and nuances. Think of it as giving your AI a well-rounded education: the broader its exposure to real-world data, the less likely it is to fill in the gaps with random nonsense. Sometimes, even a massive dataset can fail if it’s not diverse enough. Maybe it’s got plenty of English-language examples but barely any Spanish, or it only covers modern cultural references and ignores historical contexts. Developers who carefully vet their datasets—even going as far as doing incremental releases of new data—can drastically lower the odds of bizarre AI “brain farts.”
2. Offer templates or structured prompts
When AI spits out a response, it’s relying on language patterns it’s absorbed—but it doesn’t magically know the structure you prefer. By providing a clear template or format, you essentially tell the model, “This is how I want my information, no creative detours, please.” If you’re using AI chatbots like Claude or ChatGPT, you can also upload reference documents or external files. For other tools, building a retrieval-augmented generation (RAG) database can steer the AI toward reliable data sources. Let’s say you want a bulleted summary of a research paper with three key points, two recommended readings, and a concluding statement. Spell that out for the AI. The more precise you are, the fewer random tangents it will toss in. Structured guidance can filter out those last-second guesses that lead to hallucinations.
3. Limit the number of outcomes
Asking a model to produce 20, 50, or 100 examples of something can overload it, and the final entries often drop in quality. If you only need a handful of top-notch responses, limit the AI to that smaller set. It’s a bit like editing a rough draft down to the best bits—less fluff, fewer mistakes. If your marketing team wants five strong slogan ideas, don’t push for 25. A narrower request forces the AI to “think” more carefully about each response, rather than throwing out a messy pile of half-baked suggestions.
4. Test and validate
No matter how “smart” an AI system claims to be, it’s still prone to messing up. Developers need to test outputs extensively—comparing them against verified data, real-world logic, or expert input. Users, on the other hand, should approach AI’s claims with a hint of skepticism until they verify them. These tools might look brilliant one moment and spout nonsense the next. Testing helps you spot patterns in hallucinations and figure out when the model’s more likely to trip up. If you’re using AI to handle something crucial—say, medical or legal advice—you absolutely want a second opinion. Mistakes in those areas can be costly or dangerous. Consider it mandatory to test the system in a sandbox environment before letting it loose on real-world tasks.
5. Don’t substitute humans entirely
Even if you’re pulling out all the stops—fantastic datasets, templates, testing—AI can still slip. That’s where good old-fashioned human judgment comes in. Relying solely on an AI for critical decisions is a risky bet. Always cross-check what the model says against trusted sources, whether that’s an academic paper, a respected news outlet, or someone with expertise in the field. View AI outputs as conversation starters rather than definitive truths. Use them to brainstorm ideas, spark a debate, or explore new angles—but pause before running with them. If something looks off, it probably is, and double-checking won’t kill you (or your brand’s credibility).
AI hallucinations may never be fully eradicated, but you can absolutely cut them down to size. Below are some strategies that both developers and everyday AI users can employ to keep those bogus answers to a minimum. Because, let’s face it, nobody wants a chatbot telling them it’s perfectly safe to snack on rocks—or worse.
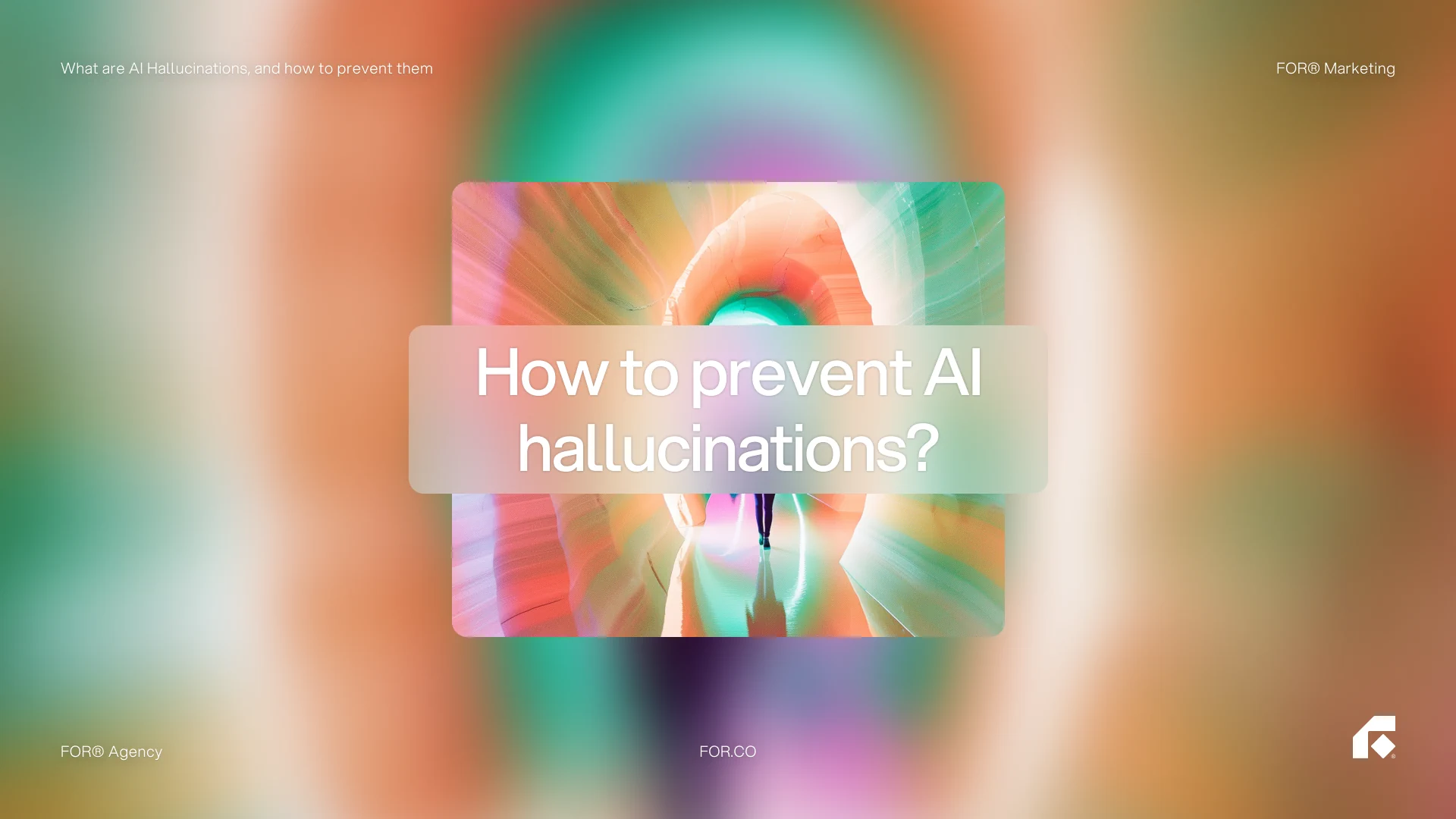
1. Improve training data quality
If your AI’s training data is missing big chunks of knowledge or skewed by bias, you’re basically handing it a recipe for half-baked results. Better, more varied data helps keep the model balanced and accurate when it encounters something new. That means bringing in comprehensive datasets that reflect a range of viewpoints and nuances. Think of it as giving your AI a well-rounded education: the broader its exposure to real-world data, the less likely it is to fill in the gaps with random nonsense. Sometimes, even a massive dataset can fail if it’s not diverse enough. Maybe it’s got plenty of English-language examples but barely any Spanish, or it only covers modern cultural references and ignores historical contexts. Developers who carefully vet their datasets—even going as far as doing incremental releases of new data—can drastically lower the odds of bizarre AI “brain farts.”
2. Offer templates or structured prompts
When AI spits out a response, it’s relying on language patterns it’s absorbed—but it doesn’t magically know the structure you prefer. By providing a clear template or format, you essentially tell the model, “This is how I want my information, no creative detours, please.” If you’re using AI chatbots like Claude or ChatGPT, you can also upload reference documents or external files. For other tools, building a retrieval-augmented generation (RAG) database can steer the AI toward reliable data sources. Let’s say you want a bulleted summary of a research paper with three key points, two recommended readings, and a concluding statement. Spell that out for the AI. The more precise you are, the fewer random tangents it will toss in. Structured guidance can filter out those last-second guesses that lead to hallucinations.
3. Limit the number of outcomes
Asking a model to produce 20, 50, or 100 examples of something can overload it, and the final entries often drop in quality. If you only need a handful of top-notch responses, limit the AI to that smaller set. It’s a bit like editing a rough draft down to the best bits—less fluff, fewer mistakes. If your marketing team wants five strong slogan ideas, don’t push for 25. A narrower request forces the AI to “think” more carefully about each response, rather than throwing out a messy pile of half-baked suggestions.
4. Test and validate
No matter how “smart” an AI system claims to be, it’s still prone to messing up. Developers need to test outputs extensively—comparing them against verified data, real-world logic, or expert input. Users, on the other hand, should approach AI’s claims with a hint of skepticism until they verify them. These tools might look brilliant one moment and spout nonsense the next. Testing helps you spot patterns in hallucinations and figure out when the model’s more likely to trip up. If you’re using AI to handle something crucial—say, medical or legal advice—you absolutely want a second opinion. Mistakes in those areas can be costly or dangerous. Consider it mandatory to test the system in a sandbox environment before letting it loose on real-world tasks.
5. Don’t substitute humans entirely
Even if you’re pulling out all the stops—fantastic datasets, templates, testing—AI can still slip. That’s where good old-fashioned human judgment comes in. Relying solely on an AI for critical decisions is a risky bet. Always cross-check what the model says against trusted sources, whether that’s an academic paper, a respected news outlet, or someone with expertise in the field. View AI outputs as conversation starters rather than definitive truths. Use them to brainstorm ideas, spark a debate, or explore new angles—but pause before running with them. If something looks off, it probably is, and double-checking won’t kill you (or your brand’s credibility).
Frequently Asked Questions
Why does ChatGPT hallucinate?
ChatGPT and similar systems revolve around predicting the next likely sequence of text. They don’t genuinely understand the meaning of your query or their own replies, so they’re bound to generate occasional nonsense.
How often does AI hallucinate?
Figures vary—some studies point to rates as low as 3% for certain models, while others note it can rise to 27%. The more unusual the question, the greater the chance for a flawed response.
Can AI ever think like a human?
At the moment, no. Current AI replays patterns based on data, while real human cognition involves conscious and unconscious processing. AI remains a powerful mimic, but it can’t fully replicate the depth of human thought.
Final Thoughts
Hallucinations highlight the present limitations of Artificial Intelligence—from small mistakes to wild confabulations. Yes, machine learning is marching forward, and developers are making progress in tackling these flaws. However, expecting AI to churn out perfect facts 100% of the time isn’t realistic just yet.
It pays to be both open-minded and critical. AI can boost productivity or spark fresh ideas, but even advanced tools like ChatGPT can mislead you with unwavering conviction. Step in with your own judgment, compare AI outputs to reliable sources, and you’ll find that these systems can be brilliant partners rather than risky solo operators.
After all, if you’re relying on a technology that can conjure misinformation from thin air, it’s best to keep one eye on the data—and the other on common sense.
Frequently Asked Questions
Why does ChatGPT hallucinate?
ChatGPT and similar systems revolve around predicting the next likely sequence of text. They don’t genuinely understand the meaning of your query or their own replies, so they’re bound to generate occasional nonsense.
How often does AI hallucinate?
Figures vary—some studies point to rates as low as 3% for certain models, while others note it can rise to 27%. The more unusual the question, the greater the chance for a flawed response.
Can AI ever think like a human?
At the moment, no. Current AI replays patterns based on data, while real human cognition involves conscious and unconscious processing. AI remains a powerful mimic, but it can’t fully replicate the depth of human thought.
Final Thoughts
Hallucinations highlight the present limitations of Artificial Intelligence—from small mistakes to wild confabulations. Yes, machine learning is marching forward, and developers are making progress in tackling these flaws. However, expecting AI to churn out perfect facts 100% of the time isn’t realistic just yet.
It pays to be both open-minded and critical. AI can boost productivity or spark fresh ideas, but even advanced tools like ChatGPT can mislead you with unwavering conviction. Step in with your own judgment, compare AI outputs to reliable sources, and you’ll find that these systems can be brilliant partners rather than risky solo operators.
After all, if you’re relying on a technology that can conjure misinformation from thin air, it’s best to keep one eye on the data—and the other on common sense.
Work with us
Click to copy
work@for.co
- FOR® Brand. FOR® Future.
We’re remote-first — with strategic global hubs
Click to copy
Helsinki, FIN
info@for.fi
Click to copy
New York, NY
ny@for.co
Click to copy
Miami, FL
mia@for.co
Click to copy
Dubai, UAE
uae@for.co
Click to copy
Kyiv, UA
kyiv@for.co
Click to copy
Lagos, NG
lagos@for.ng
Copyright © 2024 FOR®
Work with us
Click to copy
work@for.co
- FOR® Brand. FOR® Future.
We’re remote-first — with strategic global hubs
Click to copy
Helsinki, FIN
info@for.fi
Click to copy
New York, NY
ny@for.co
Click to copy
Miami, FL
mia@for.co
Click to copy
Dubai, UAE
uae@for.co
Click to copy
Kyiv, UA
kyiv@for.co
Click to copy
Lagos, NG
lagos@for.ng
Copyright © 2024 FOR®
Work with us
Click to copy
work@for.co
We’re remote-first — with strategic global hubs
Click to copy
Helsinki, FIN
hel@for.co
Click to copy
New York, NY
ny@for.co
Click to copy
Miami, FL
mia@for.co
Click to copy
Dubai, UAE
uae@for.co
Click to copy
Kyiv, UA
kyiv@for.co
Click to copy
Lagos, NG
lagos@for.ng
Copyright © 2024 FOR®